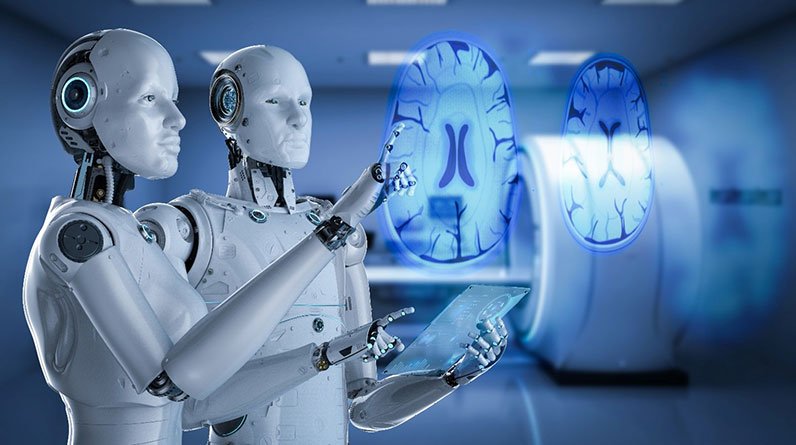
AI and Radiology Health and X-Ray
Radiology, a pivotal discipline in modern medicine, revolves around the use of medical imaging techniques to diagnose and monitor various health conditions. From X-rays and CT scans to MRI and ultrasound, radiological imaging provides invaluable insights into the inner workings of the human body.
In recent years, the integration of artificial intelligence (AI) has brought a transformative shift to the field of radiology, enhancing the accuracy, speed, and efficiency of image analysis and, consequently, patient care.
Understanding AI and Machine Learning Algorithms
At its core, AI is a branch of computer science that empowers machines to perform tasks that typically require human intelligence. Machine learning, a subset of AI, involves the development of algorithms that enable systems to learn and improve from experience without being explicitly programmed.
In the context of radiology, machine learning algorithms excel in recognizing patterns within medical images. They accomplish this by training on large datasets of annotated images and learning to identify features associated with specific conditions.
AI Integration in Radiology
The fusion of AI and radiology has introduced a new era of diagnostic precision and efficiency. AI algorithms, armed with their ability to rapidly analyze vast amounts of image data, excel in automating routine tasks.
In radiology, this translates to the swift detection of anomalies such as tumors or fractures that might otherwise require painstaking human scrutiny. AI algorithms can also aid radiologists by providing a preliminary analysis, highlighting potential areas of concern, and prioritizing cases based on urgency.
This collaborative approach optimizes the radiologist’s workflow, allowing them to focus on nuanced diagnoses and decision-making.
Benefits of AI in Radiology
Radiology has experienced many transitions with the integration of AI technologies.
This convergence has been extremely beneficial to the field of radiology, enhancing the capabilities of radiologists and improving patient care.
The following is an elaboration on these benefits:
Increased Efficiency
AI has fundamentally changed the way radiologists interpret and analyze medical images, significantly boosting their workflow efficiency. AI algorithms have the remarkable capacity to swiftly process and analyze the information obtained from these radiological pictures and help provide diagnoses.
This acceleration in diagnostic capacity allows for additional patient evaluation in an expedited manner. It also allows radiologists to focus their expertise on making informed decisions rather than dedicating excessive time to routine tasks.
Consequently, healthcare institutions can accommodate a higher volume of cases while maintaining a streamlined workflow.
Improved Accuracy
The precision and accuracy of diagnostic outcomes have witnessed a notable advancement with the incorporation of AI in radiology. AI algorithms that are trained on extensive datasets have the quality of never forgetting information, and the more information the machine learns, the better it becomes at recognizing details that can elude even the keenest human eye.
It’s also important to note that this heightened sensitivity translates to the ability to detect conditions at earlier stages, enabling prompt interventions and better patient outcomes.
Cost-Effectiveness
AI-driven radiology solutions also bring an element of cost-effectiveness to healthcare systems.
Put simply, the automation of image analysis and preliminary reporting reduces the burden on radiologists and allows them to allocate their time more strategically. Timely and accurate diagnoses facilitated by AI can lead to efficient treatment planning, shorter hospital stays, and reduced healthcare costs in the long run.
Enhanced Patient Care
Finally, at the heart of the healthcare journey lies patient care, and AI in radiology enhances this aspect profoundly. Patients benefit from reduced wait times for results, leading to a faster initiation of treatment plans. Additionally, the improved accuracy of AI-driven diagnostics means fewer instances of misdiagnosis or delayed diagnoses, resulting in more tailored and effective treatment approaches.
Examples of AI in Image Analysis
1. Detecting Lung Cancer from Chest X-rays
AI’s proficiency in analyzing medical images has proven instrumental in detecting lung cancer from chest X-rays. Machine learning algorithms can be trained on an extensive dataset of X-ray images, learning to identify subtle indicators of cancerous growths.
This technology excels at highlighting abnormalities that might escape the human eye, allowing radiologists to swiftly identify potential cases of lung cancer. By expediting the identification of cancerous lesions, AI not only improves diagnostic accuracy but also contributes to the timely initiation of treatment, potentially saving lives.
2. Identifying Fractures or Bone Abnormalities
The rapid and accurate identification of fractures and bone abnormalities is another arena where AI shines. Machine learning algorithms trained on diverse images of bones can swiftly detect fractures, even in cases where they might be obscured by surrounding tissue.
These algorithms are capable of processing a wide range of fractures, from subtle hairline cracks to more complex breaks. By automating the fracture detection process, AI accelerates diagnosis, enabling prompt interventions and reducing the risk of complications.
3. Detecting Breast Cancer from Mammograms
Finally, AI’s application in mammography has introduced a groundbreaking approach to breast cancer detection. Machine learning algorithms can analyze mammograms to identify potential areas of concern, including masses, microcalcifications, and other anomalies.
AI’s ability to learn from an extensive array of mammogram images enables it to recognize patterns associated with breast cancer, helping radiologists prioritize cases for further evaluation. By providing a reliable second opinion, AI enhances the sensitivity of breast cancer detection, ultimately leading to earlier diagnosis and improved treatment outcomes.
Final Thoughts
Ultimately, AI’s contributions to radiology foster a healthcare environment where patients receive quicker, more accurate diagnoses and more personalized care. The synergy between human expertise and AI-driven efficiency and accuracy amplifies the capabilities of radiologists, streamlines workflows, and ultimately advances patient care.